CALIBRATION & PERFORMANCE BENCHMARKING
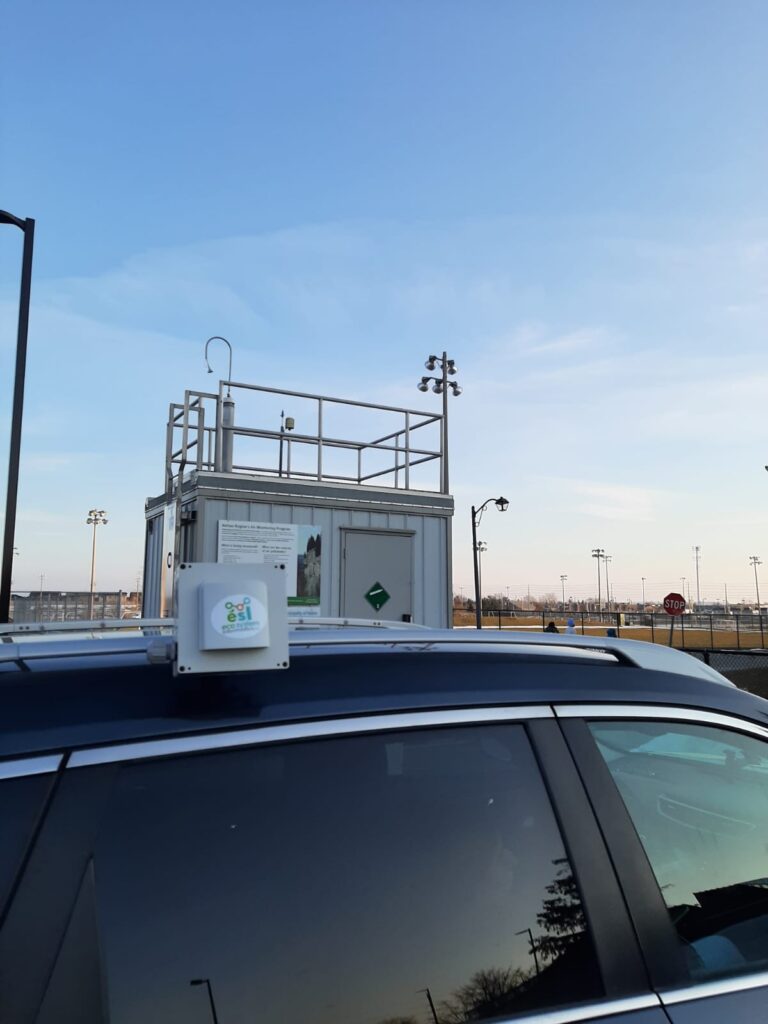
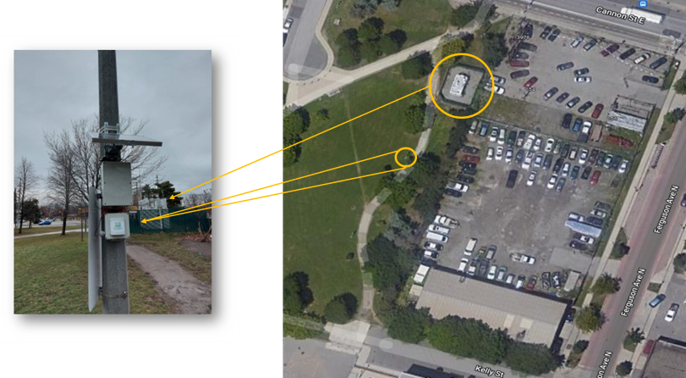
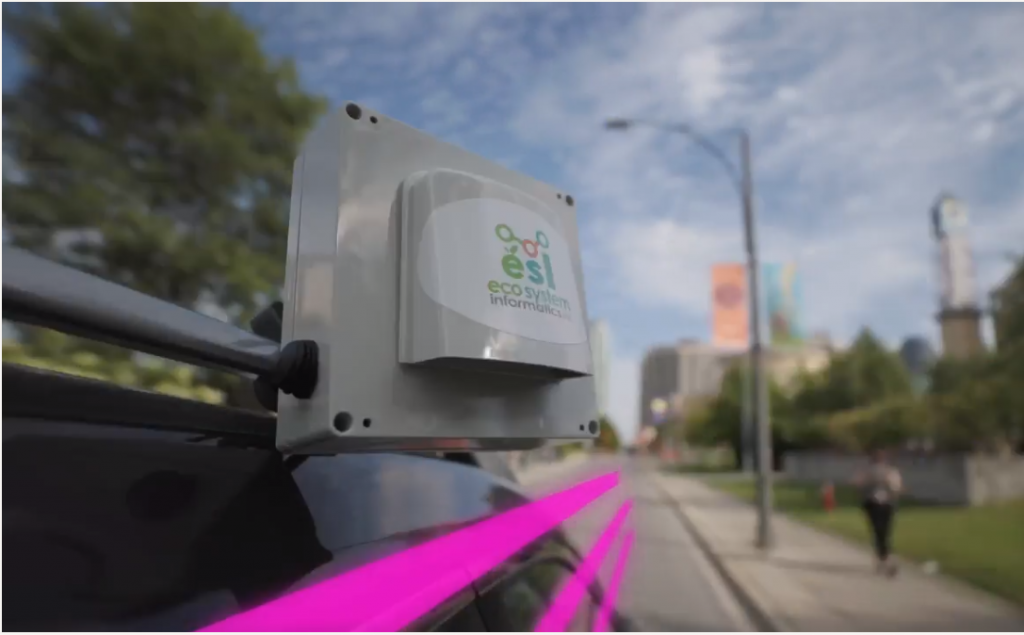
The foundational principles of air quality measurement remain the same across all platforms, however the distinguishing feature of ESI’s technology lies in its calibration capabilities.
Why Calibration matters?
All sensors, irrespective of their make, are prone to inaccuracies due to a multitude of factors—be it environmental changes, vibrations, or simply wear and tear. Calibration serves to correct these inaccuracies, ensuring that the sensor data you rely on is as accurate and reliable as possible.
What sets ESI apart?
ESI devices are not just compact and versatile; they offer an added layer of accuracy through continuous, AI-based calibration. This feature negates the need for bulky, resource-intensive hardware, which is a requirement in traditional air quality monitoring equipment.
To illustrate this leap in technology, we present a comprehensive performance benchmarking of ESI’s devices against a government-run measurement station.
ESI’s modular measuring device accommodates various sensor combinations, enabling a wide range of gas concentration measurements. Specifically, our focus for this benchmarking study includes the following sensors:
- Carbon Monoxide (CO)
- Nitrogen Dioxide (NO2)
- Ozone (O3)
- Sulphur Dioxide (SO2)
- Particulate Matter (PM 2.5)
Additionally, we have also examined the device’s performance in both fixed (stationary) and mobile unit modes.
Stationary/Fixed Mode Calibration
Our test unit was stationed next to a Government measurement station in the City of Hamilton, Ontario, to collect data for a period of nearly 1 year, spanning three different seasons. This data was then calibrated using ESI’s proprietary AI algorithms and compared to the reference data.
Mobile Mode Calibration
The Game Changer: Mobile Calibration
ESI devices break the mold by offering unparalleled performance in both stationary and mobile environments. The AI-driven calibration adapts seamlessly to varying conditions due to the movement, which is an industry-first feature.
Methodology: Keeping it Mobile
For this phase of the study, ESI devices were mounted on a moving vehicle within the City of Hamilton, Ontario. Data was then collected over 19 scan days across 6 months. The nearest reference was the Hamilton Downtown station located in Beasley Park. Data from the ESI device was filtered whenever the device was within approximately 200m of the government reference station, and this subset was used to train and test the AI calibration algorithms.